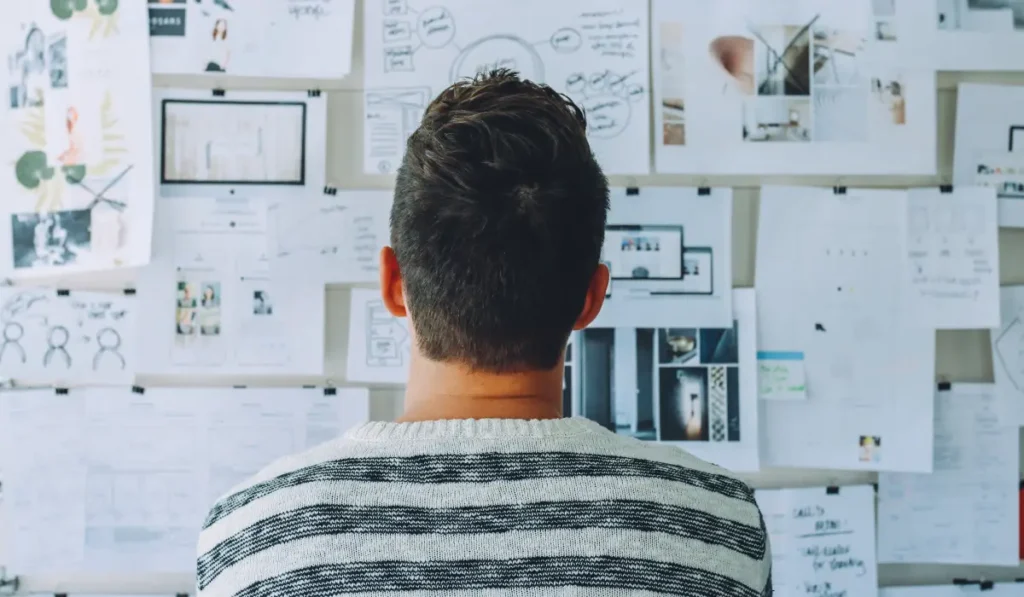
What is an AI Strategy?
- Product-Level: Tied to a specific application, feature, or service.
- Business Unit-Level: Scoped to a department or function.
- Organizational-Level: Cross-functional and enterprise-wide.
- AI Startup Strategy: Centered around AI-native products and business models.
-
We will discuss these scopes later in the blog, but before that, understand why you need an AI strategy framework (in case you have not considered it yet).
Why Do Businesses Need an AI Strategy?
Deploying AI without a clear strategy can quickly lead to wasted resources, misaligned initiatives, and scaling challenges. AI projects that lack strategic direction may not address the real business problems, resulting in inefficiencies and unmet expectations.
For example, one of our retail clients launched an AI tool to offer personalized recommendations to their customers. However, the project struggled due to a lack of data preparation, something very crucial for any AI initiative. They didn’t ensure their data was clean, structured, or easily accessible. The result? The AI system couldn’t generate accurate recommendations, leading to poor customer engagement. This is a clear case where a lack of upfront strategy and data readiness led to avoidable setbacks.
When AI initiatives aren’t part of a broader project, businesses focus on the wrong solutions and overinvest in tools that don’t align with their long-term objectives. As a result, the AI projects may not deliver significant value, and scaling them becomes more difficult. Similarly, skipping over important factors like compliance or future-proofing the technology can result in costly changes down the road.
On the Other Hand, AI Strategy Framework Ensures
- All priorities are well-defined. It starts by defining AI projects that have a valuable impact and are possible to implement.
- Your resources and teams are aligned across initiatives.
- Infrastructure, data, and skills are evaluated before deployment.
- Risk, compliance, and accountability structures are defined upfront.
- Organizations pursuing AI as a horizontal capability rather than a set of disconnected pilots require a strategy tied to transformation goals.
The Four Scopes of AI Strategy: Where Do You Fall?
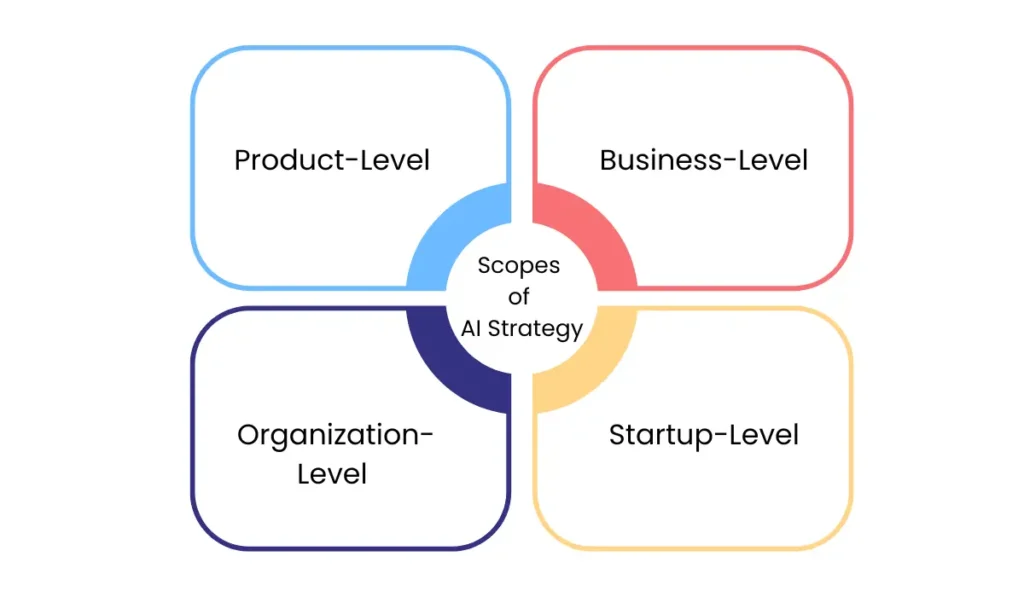
1. Product-Level AI Strategy
If you have an AI product or are planning to develop one, you need a product-level AI strategy. It focuses on embedding AI within a discrete application, feature, or service. The objective is to improve product functionality, automate user interactions, or introduce data-driven capabilities that enhance the product’s value proposition.
Product managers typically own this strategy with data science and engineering teams. It applies when AI is a feature enhancement, not a core business model.
Use cases could be recommendation engines, intelligent search, personalization layers, or image classification in digital health apps.
Execution Focus
- Data Readiness: Ensure high-quality, well-labeled data is available for model training. Fragmented or data without structure should be reconsidered, or the implementation should be paused.
- Technical Resourcing: Assign cross-functional teams with clear responsibilities across data pipelines, model development, and system integration.
- Feedback Loops: Design telemetry and logging mechanisms to monitor model performance and enable post-deployment retraining.
- Infrastructure: Validate that existing tech stacks (e.g., APIs, databases, MLOps tools) support the full AI model lifecycle.
- Metrics Alignment: Define success criteria for product KPIs, such as feature usage, conversion rate, or cost-to-serve reduction.
- Experts Tip: Do not proceed with model development if you are unsure about data ownership, consistency, or volume. Also, prioritize feature robustness over model complexity.
2. Business Unit-Level AI Strategy
A business unit-level AI strategy targets functional areas such as sales, finance, supply chain, or customer service. The goal is to apply AI to improve business outcomes within the scope of the unit, such as cost optimization, risk reduction, or revenue acceleration.
Ownership typically resides with the business unit head, supported by an AI enablement or analytics team. This level is ideal when functional performance metrics can be directly tied to AI intervention.
Execution Focus
Successful execution of AI strategy for your business depends on aligning use cases with the unit’s objectives and operational realities.
- Use Case Prioritization: Apply a value-feasibility matrix to identify high-impact opportunities with accessible data. Avoid projects for which you have limited or unclear data and business metrics.
- Manual Baselines: In low-data environments, implement rule-based systems or human-in-the-loop processes to simulate future AI behavior and generate training data.
- Cross-Functional Input: Engage process owners early to define operational parameters and avoid downstream resistance.
- Technology Fit: Choose tools that integrate with the unit’s existing systems (e.g., CRM, ERP). Avoid standalone models without workflow integration.
- Performance Monitoring: Link AI output to P&L metrics, such as cost savings, process throughput, or customer satisfaction.
Experts Tip: Do not introduce AI as a technical experiment. It must be scoped as a business initiative with defined change management and accountability structures.
3. Organizational-Level AI Strategy
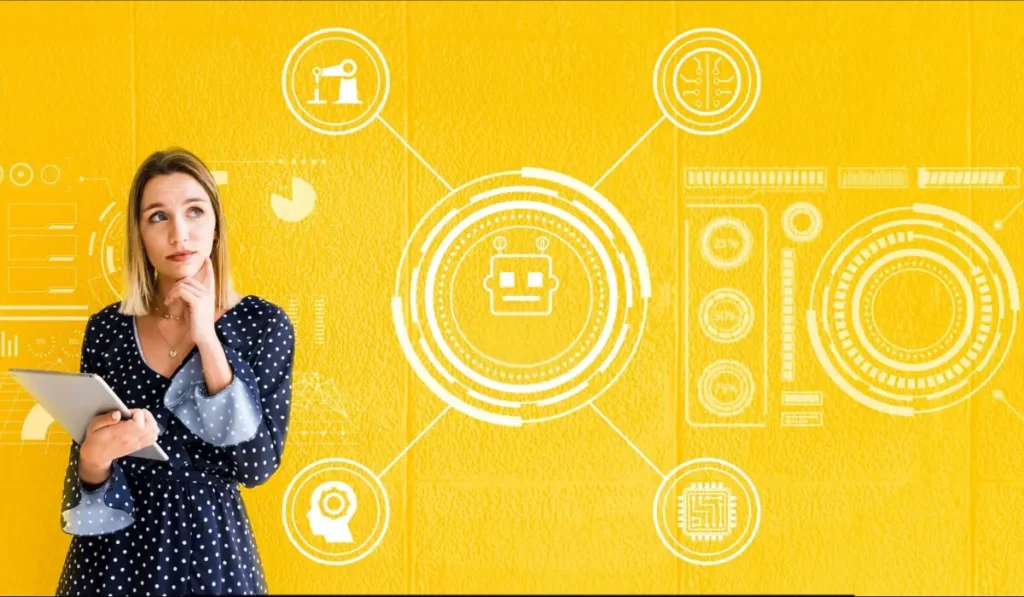
An organizational-level AI strategy spans the enterprise. It aims to embed AI as a horizontal capability that drives innovation, operational efficiency, and strategic differentiation. This strategy, owned by the CIO, Chief Data & Analytics Officer, or Chief Digital Officer, requires alignment across business functions, IT, risk, and compliance.
This approach is essential when AI is central to the corporate transformation agenda or is expected to support multiple, scalable use cases.
Execution Focus
- Budget: Establish centralized or federated funding mechanisms for AI initiatives. Allocate sufficient resources for ongoing experimentation and long-term scaling of AI initiatives.
- Culture: Invest in change management. Build trust in model outputs by ensuring transparency, explainability, and clear accountability.
- Infrastructure: Deploy enterprise-grade MLOps and data infrastructure to support reusability, monitoring, and model governance at scale.
- Data: Implement unified data governance policies, metadata standards, and data quality controls across the organization.
- Skills: Address capability gaps through targeted hiring, reskilling programs, and external partnerships.
Pay more attention to:
- Governance Models: Define ownership structures, whether centralized through a Center of Excellence (CoE) or distributed in a federated model, with clear guardrails for compliance and performance monitoring.
- Risk Management: Integrate AI into enterprise risk frameworks, covering model bias, fairness, cybersecurity, and regulatory exposure.
- Experts Tip: Without foundational capabilities (especially data and culture), scaling AI beyond pilots will not bring sustained business value. So, begin with lighthouse projects that validate the operating model and governance structure.
4. AI Startup Strategy
An AI startup strategy is purpose-built for companies whose core product or service is powered by AI. Unlike enterprises, which adopt AI incrementally, startups must build AI into their value proposition from day one.
The strategy typically belongs to the founder or product leadership. It must address technical capability, market differentiation, trust, and scalability.
Execution Focus
- Model Performance: Prioritize early development of high-performing models with reproducible benchmarks. .
- Data Acquisition Strategy: Design the product to continuously collect proprietary data. This supports long-term defensibility and model improvement.
- User Trust and UX: To support customer adoption and regulatory compliance, include explainability, fallback behaviors, and privacy by design.
- Go-to-Market Alignment: Ensure AI capability is articulated in business terms (e.g., faster decisions, better outcomes), not just technical novelty.
- Feedback Infrastructure: Build mechanisms to ingest user feedback, errors, and edge cases for ongoing model refinement.
- Experts Tip: Speed-to-market should not compromise model validation. Investors, partners, and customers will evaluate business value and model governance.
4 Steps to Build an AI Strategy for Your Business
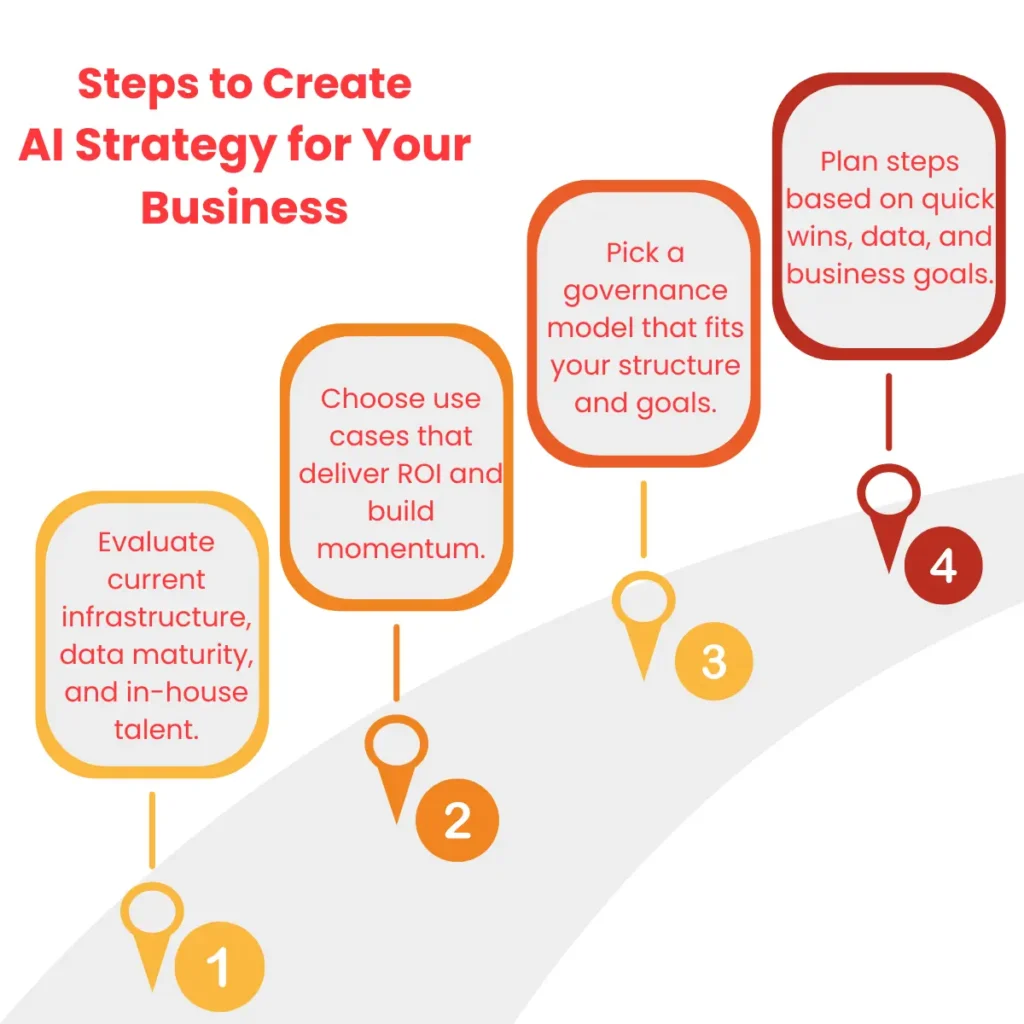
- Run a Capability Gap Assessment: Evaluate infrastructure, data, and talent against the intended level of strategy.
- Select Strategic Use Cases: Use prioritization matrices that consider both business impact and feasibility.
- Establish Operating Models: Decide between centralized, federated, or hybrid governance structures.
- Develop a Roadmap: Sequence initiatives based on quick wins, data readiness, and alignment with transformation programs.
Need Help? Experts are on the Way!
- Refine your AI product strategy to meet market demands and deliver measurable outcomes.
- Optimize model performance and ensure your AI tools continuously improve and meet user expectations.
- Mitigate risks associated with AI adoption and build trust with your customers.
Ready to take the next step in developing a winning AI strategy? Contact us today.